
Since the launch of Chat GPT, AI has been the two-letter acronym on everybody’s lips. It took no time at all for large language models (LLMs) to proliferate across the economic spectrum but the final frontier – and that which arguably has the most to gain – is the public sector, writes Nick Slater.
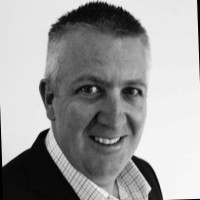
A recent survey conducted by the Australian Public Service Commission found Australians saw many potential benefits from using AI in public services with quicker and more efficient provision of public services, cost savings, personalisation, and increased accuracy chief amongst them.
As the Australian Government considers the best way to introduce AI into the public service, there is a delicate balancing act it must navigate between two seemingly diametrically opposed factors.
On the one hand, to reap the promised benefits of AI, more public servants must be given greater access to government data. On the other, strong data governance is required to ameliorate the risks around privacy, security, and data quality that this wider data sharing presents.
What we’ve seen in the private sector is that the enterprises which balance these seemingly opposing trends — democratising data, while maintaining strong governance over that data — are those who see the greatest success in their AI strategies.
Achieving equilibrium
For the Federal Government, achieving this equilibrium boils down to answering one key question: how do you implement comprehensive governance controls without stifling innovation?
The first step is to unify data in a central location that multiple teams from different agencies can access easily and securely. Everything from weather predictions, census data, financial information, property ownership records, demographic trends, employment statistics, energy production, and much more is collected and stored by one agency or another, often on a mixture of disparate data storage platforms. Removing silos and unifying data allows governance to be centralised and applied consistently, while simultaneously minimising complexity and optimising costs.
After this foundation has been implemented, traditional governance practices that apply in any environment become even more critical as generative AI increases access to data across the public sector.
Fine-grained control policies are foremost among them. As more people gain access to more data, the potential for personally identifiable information (PII) to be leaked or seen by the wrong users increases. These policies, together with anonymisation and de-identification techniques, are key to keeping data private.
There will be cases in which employees may want to examine a data set outside of their direct access policy. This can be easily overcome when data is stored in a central location as ‘differential privacy’ can allow users to share and explore the patterns within data sets, but without revealing any individual’s PII.
‘Data clean rooms’
Taking this a step further, ‘data clean rooms’ allow multiple parties to collaborate on data without actually disclosing the raw data to one another. These are typically used to share data between different organisations in the private sector, but there is an emerging trend towards the technology being used internally to meet increasing regulatory and privacy needs as it provides additional protection for PII data.
While these controls prevent misuse – whether intentional or accidental – from internal users, data must also be safeguarded from external threats, particularly when that data is as sensitive as the information held by the Federal Government.
As such, security must be built into the fabric of any centralised data platform rather than trying to bolt it on later. If two or more systems are keeping track of who can access what data, for example, the chance of errors and unauthorised access greatly increase.
Specific technologies that can play a key role in securing data for generative AI include continuous risk monitoring and protection, role-based access control (RBAC), and granular authorisation policies.
Breaking down data silos
Ultimately, the major issue which needs to be prioritised in the pursuit of AI is breaking down the data silos that impact decision making. It is common for people to think of their national government as a singular entity but, in reality, it is a federation of independent agencies and departments. This fragmentation is not unique to Australia.
A single platform that allows these data sets to be shared securely between agencies will enable AI to truly deliver on its promise.
So, before the Government implements anything resembling an ‘AI strategy’ it first needs to have a ‘data strategy’.
Effective data management is the first step before AI can unlock the information needed to serve the public good. After all, without data, there is no AI.
*Nick Slater is Senior Regional Director – Public Sector, Snowflake ANZ
Comment below to have your say on this story.
If you have a news story or tip-off, get in touch at editorial@governmentnews.com.au.
Sign up to the Government News newsletter